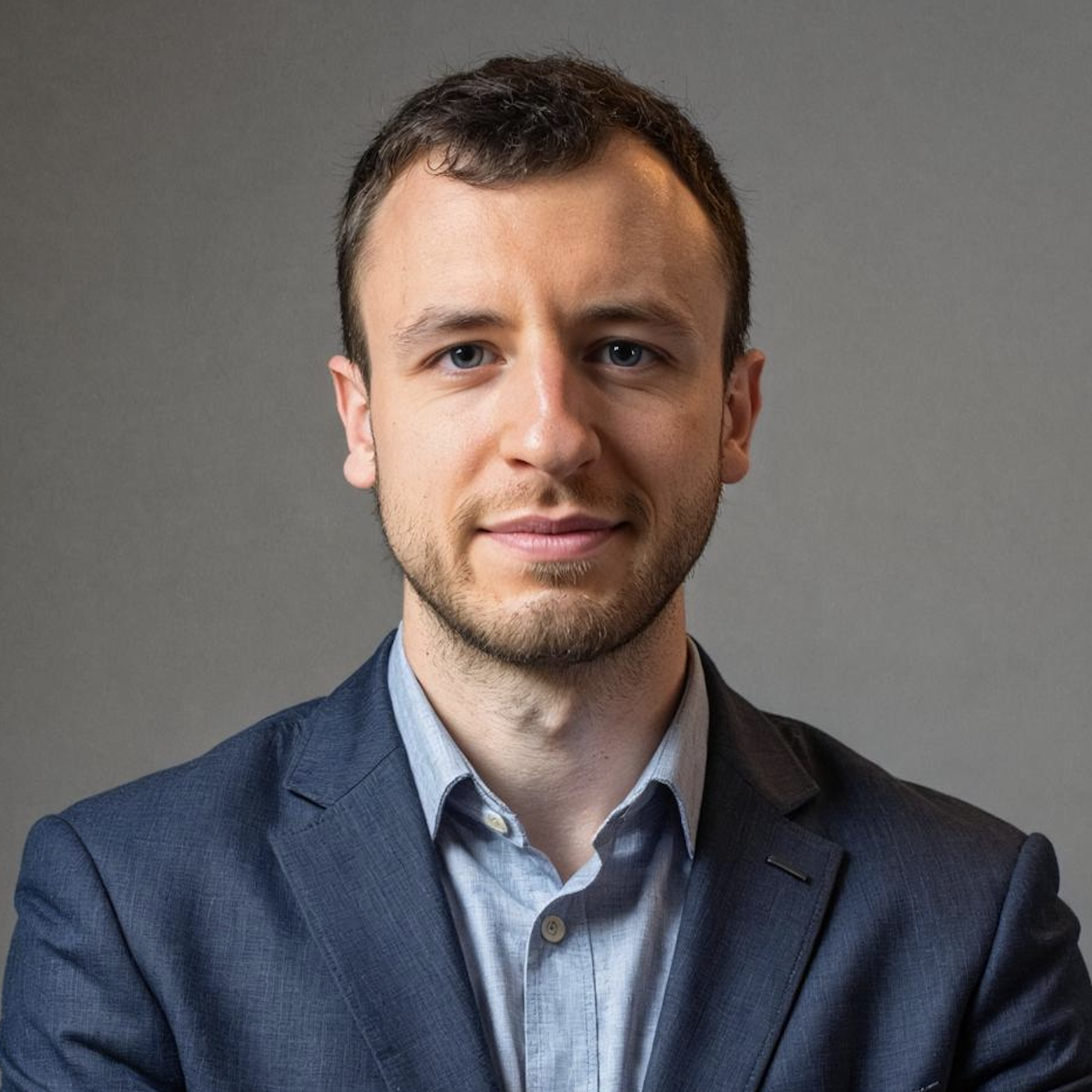
In the past I also did work in cryptography and pure mathematics, including number theory, algebraic geometry, and geometric measure theory. Fun fact: I actually started grad school as a PhD student in Brown's math department, conducting research in analytic number theory and cryptography with Jeff Hoffstein. I've since switched to AI, but I still like to make my background in pure math useful in my AI research. After getting my masters degree in mathematics in spring 2022, I took a professional leave of absence for a year to gain exposure to ML in industry. I did three internships: at American Express AI Labs , Akkio (a no-code AI startup), and Captions (an AI video editing startup).
I completed my undergraduate degree at Wesleyan University. During my time in college I spent one semester at the Math in Moscow program and another at the Budapest Semesters in Mathematics program. My undergraduate math research advisor was Ken Ono, I spent two summers doing research with him at Emory University's Research Experience for Undergraduates.
I'm particularly inspired by the life of Walter Pitts, who proposed the first mathematical model of the neural network.
nate_gillman [at] brown.edu
Publications (AI/ML)
2025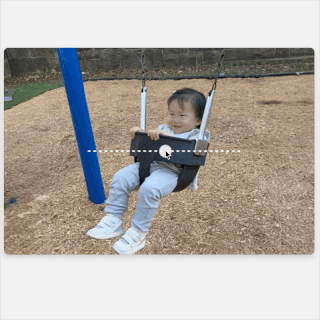
Nate Gillman, Charles Herrmann*, Michael Freeman, Daksh Aggarwal, Evan Luo, Deqing Sun, and Chen Sun*.
arXiv / Code / Project Page
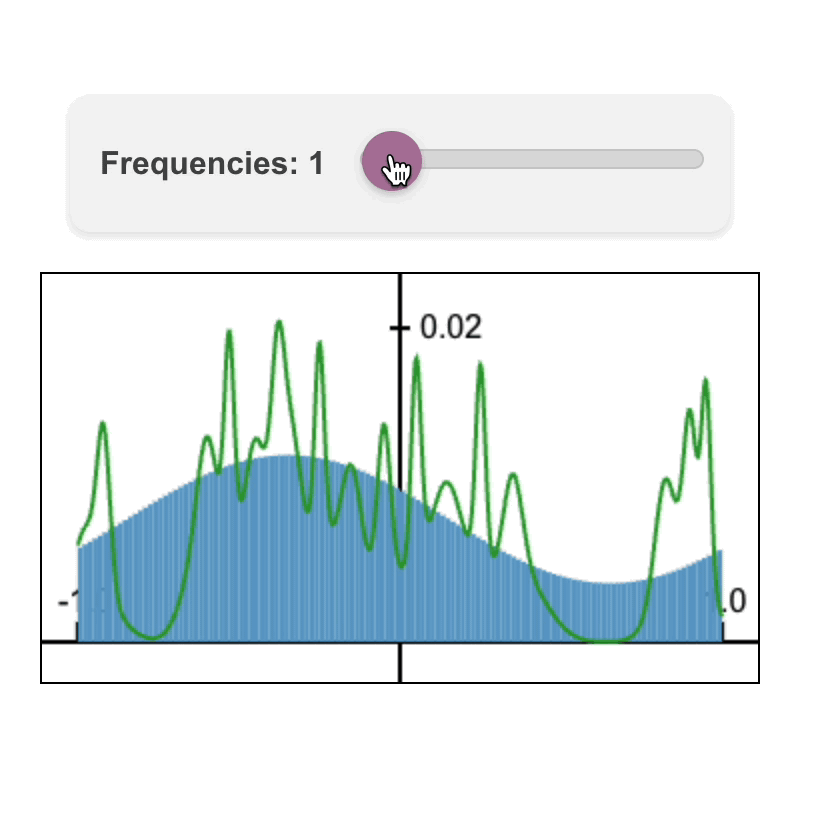
Nate Gillman*, Daksh Aggarwal*, Michael Freeman, Saurabh Singh, and Chen Sun. ICLR 2025.
arXiv / Code / Project Page
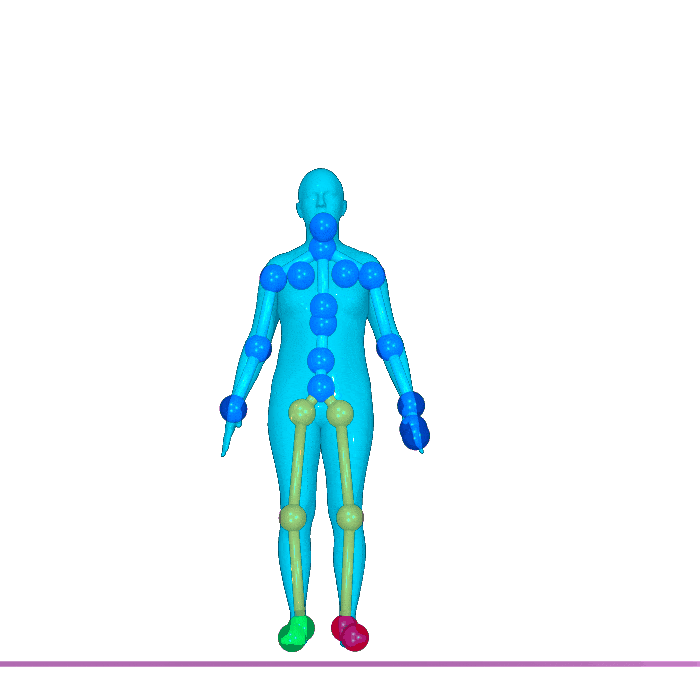
Nate Gillman, Michael Freeman, Daksh Aggarwal, Chia-Hong Hsu, Calvin Luo, Yonglong Tian, and Chen Sun. ICML 2024.
arXiv / Code / Project Page / Conference
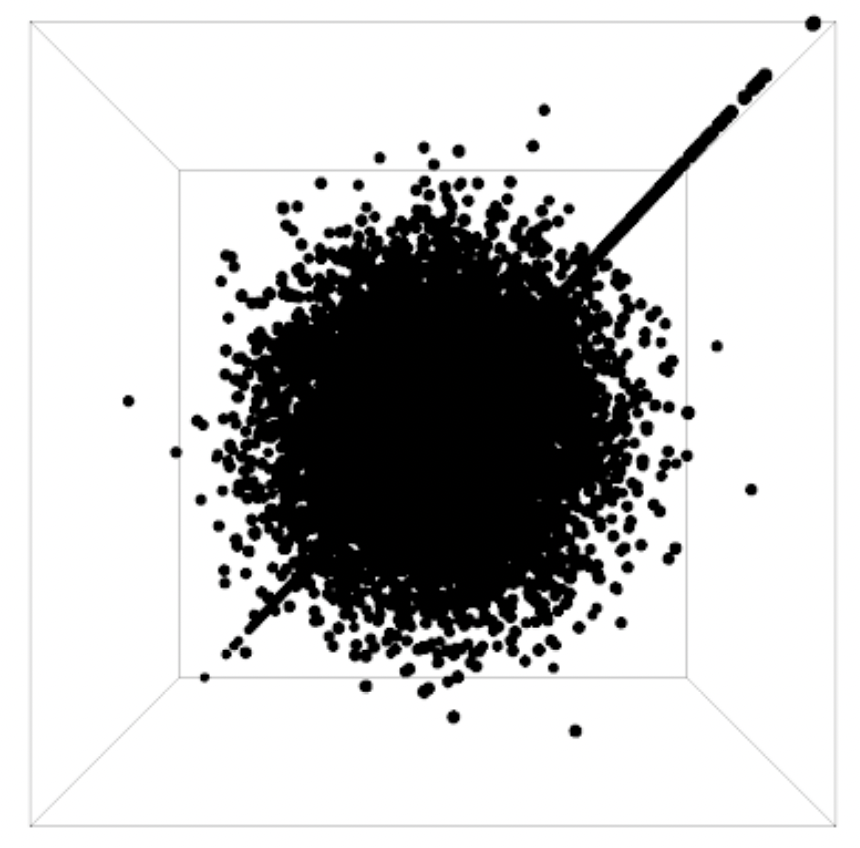
William Rudman, Nate Gillman, Taylor Rayne, and Carsten Eickhoff. ACL 2022.
arXiv / Code / Conference
Patents (AI/ML)
2022
Methods and systems for automatically generating and executing computer code using a natural language description of a data manipulation to be performed on a data set
Nate Gillman, Nadia Laflaf, Abraham Parangi, Jonathon Reilly, and Nathan Wies. U.S. Patent Application No. WO 2024/073098 A1. Filed Sep 29, 2023.
Nate Gillman, Nadia Laflaf, Abraham Parangi, Jonathon Reilly, and Nathan Wies. U.S. Patent Application No. WO 2024/073098 A1. Filed Sep 29, 2023.
Publications (Mathematics)
2021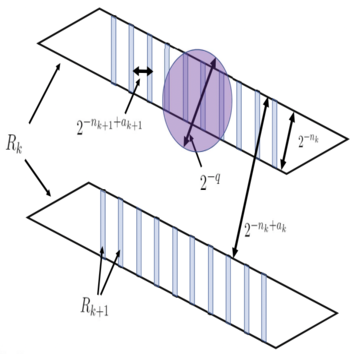
Frank Coen, Nate Gillman, Tamás Keleti, Dylan King, and Jennifer Zhu (2021). Annales Fennici Mathematici, 46(2), 683-702.
arXiv / Journal
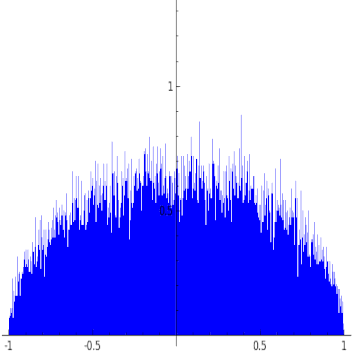
Nate Gillman, Michael Kural, Alexandru Pascadi, Junyao Peng, and Ashwin Sah (2020). Research in Number Theory, 6(9).
arXiv / Journal / MathSciNet
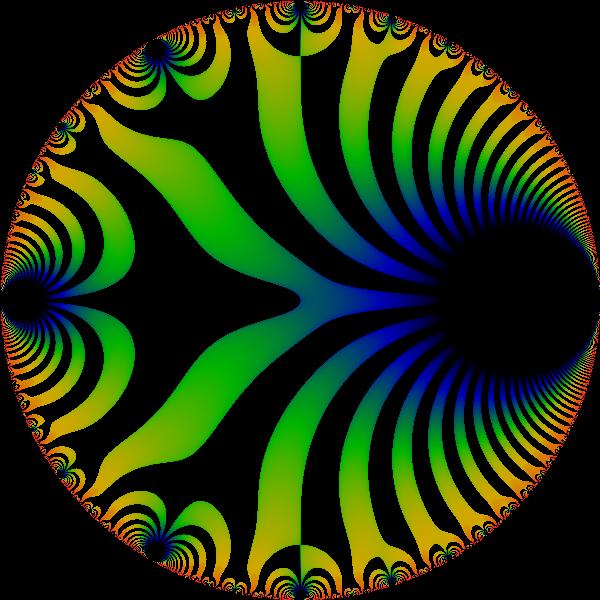
Nate Gillman (2020). Journal of Number Theory, 206, 46-61.
arXiv / Journal / MathSciNet
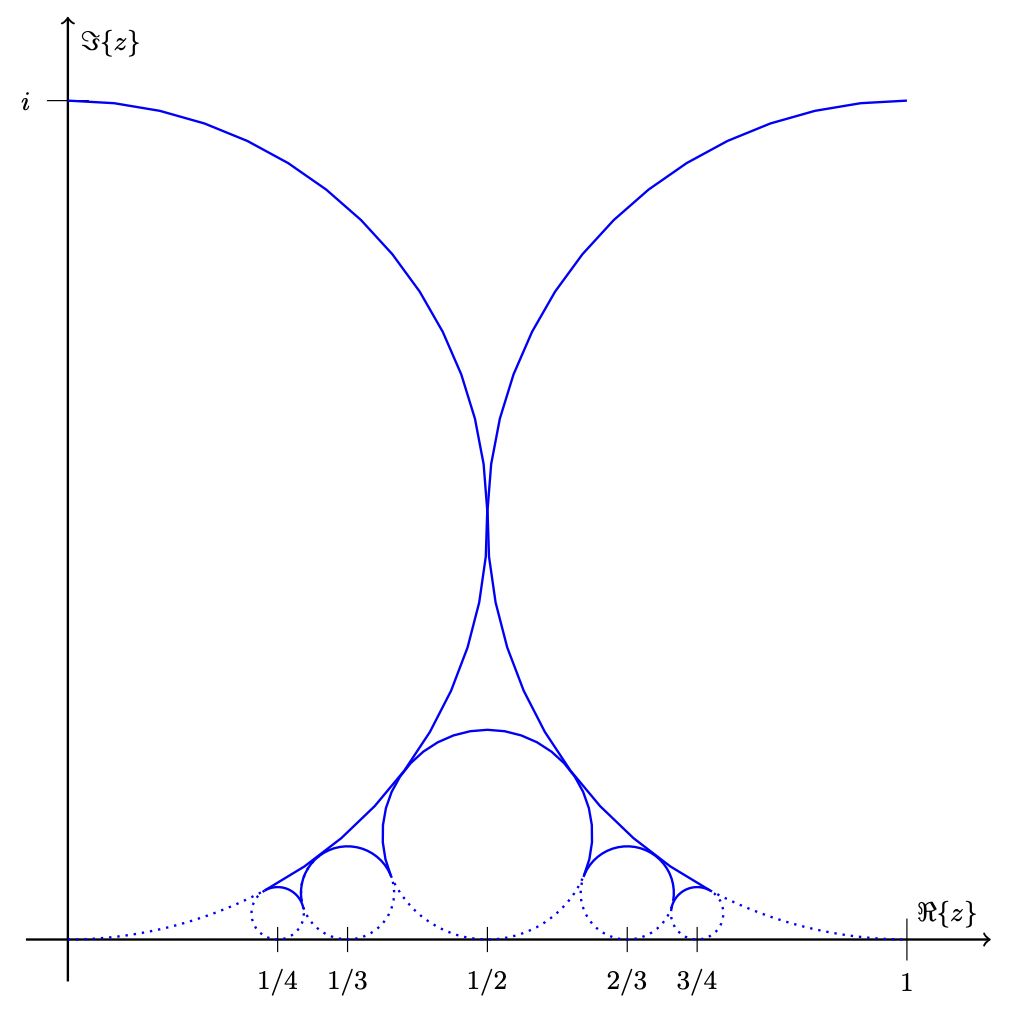
Nate Gillman, Xavier Gonzalez, Ken Ono, Larry Rolen, and Matthew Schoenbauer (2019). Philosophical Transactions of the Royal Society A, 378: 20180435.
arXiv / Journal / MathSciNet
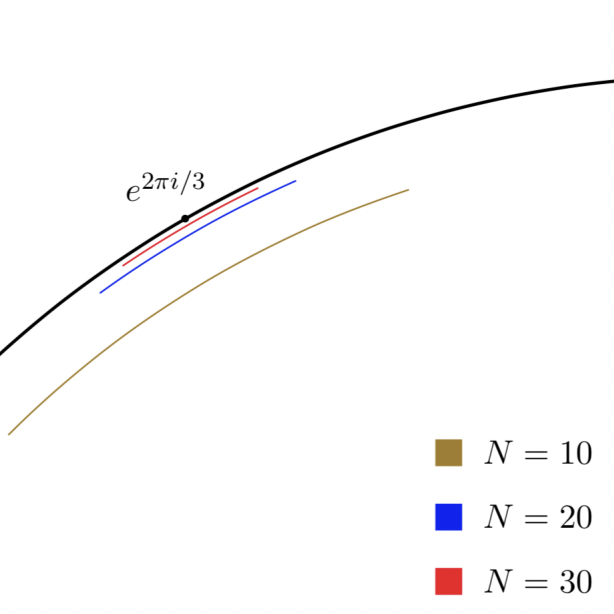
Nate Gillman, Xavier Gonzalez, and Matthew Schoenbauer (2018). Research in Number Theory 4(39).
arXiv / Journal / MathSciNet
Updates
- [Oct-2024] Our arXiv preprint proposes a new neural network layer, the Fourier head, which learns a continuous probability density function using Fourier series, and returns a discrete approximation of it. When to use it? Large language models are often adapted to model non-linguistic tokens. If these tokens have an underlying continuous structure, then replacing the linear classification head with the Fourier head can boost downstream performance. You can find code and visuals on our project page.
- [Feb-2024] Our arXiv preprint aims to stabilize self-consuming generative model training. We support our proposed method with rigorous proofs, as well as experiments on the challenging human motion synthesis task. You can find human motion visuals and code on our project page.